Podcast: All The Brain Chip Implant Benefits & None of The Risks
In this episode, we discuss how a team from Carnegie Mellon University spearheading non-invasive brain computer interface solutions has had a significant breakthrough in improving their accuracy.
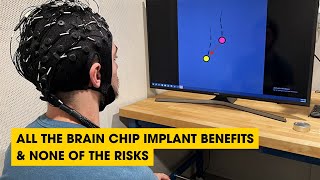
In this episode, we discuss how a team from Carnegie Mellon University spearheading non-invasive brain computer interface solutions has had a significant breakthrough in improving their accuracy.
EPISODE NOTES
(0:50) - All The Brain Chip Implant Benefits & None of The Surgery
Become a founding reader of our newsletter: read.thenextbyte.com
Transcript
Hey friends, in today's episode, we're putting a PSA out there. If you've seen all the news about Elon Musk's Neuralink company drilling holes in people's head to put a chip in there, stop, don't do it. This team from Carnegie Mellon is doing something really interesting using AI to make sure that non-invasive BCIs, which don't require surgery, work just about as well, give you all the benefits without the hole in your head. Let's jump in and check it out.
I'm Daniel, and I'm Farbod. And this is the NextByte Podcast. Every week, we explore interesting and impactful tech and engineering content from Wevolver.com and deliver it to you in bite sized episodes that are easy to understand, regardless of your background.
Daniel: What's up friends? Today we're talking about a way that you can reap all of the brain chip implant benefits that come along with something like Neuralink, but without having to drill a quarter sized hole in your skull. And we've talked about Neuralink before in the podcast, and we've talked a little bit about brain computer interfaces, but it's as a primer, essentially the idea is that you can use your brain to control a computer.
Farbod: It's a perfect solution for all of our listeners out there that have commitment issues, right? You get all of the goodies.
Daniel: You have to explain this to me.
Farbod: You get all of the goodies of a brain computer interface without any of the commitment to a chip in your head. None of the surgery, none of that.
Daniel: I see where you're going there. It took me a second to catch up, but I get it. I agree, right? So, kind of the background here, traditional brain computer interfaces that, again, allow you to control a computer or device using your brain signals is really, really awesome for folks with certain types of medical conditions. I think one of the most textbook examples is like you're paralyzed and you're unable to control a computer mouse and you're unable to communicate. So how can we use signals in your brain that is still intact, even though maybe the neural pathways to the rest of your body are not? How can we use the signals in your brain that are still intact to help you to be able to communicate, help you to be able to control things around you. And I think the ultimate end state there is like, maybe someone who's a paraplegic could control a robotic exoskeleton and be able to walk around and return people's ability to move and to walk. I think generally here, the motivation is pretty noble, let's say. Like it's meant to help a lot of people. And honestly, like for things like Neuralink, which is this company that Elon Musk helped found, they had recent trials in January, human trials. The results came out in February saying like, it's awesome. This person can control a computer cursor using their brain. They just started clinical trials on a next batch of patients. Again, many of them have these conditions where they would benefit from this neural implant, clinical trials in Phoenix started yesterday as, as of the time we're recording this, which is crazy. But again, the way that these devices are usually set up, involve a really invasive brain surgery, which can be really, really risky and limit your use. So, I mean, again, the drawbacks there being, there are all the risks that come along with having an open brain surgery, and what if it doesn't work, or what if it doesn't work as well as you hoped, or like you're saying, if you've got commitment issues and you're not ready to commit to having your brain cut open, what's the happy medium here? What's something that we can allow people without having a hole cut in their skull to implant neuro-nano electrodes in their brain. What's the solution that we can provide knowing that technology does exist through EEGs to read brain waves, to read brain activity without having to drill into the skull and contact the brain.
Farbod: And you know what, before we dive into the conversation, I wanna preface it by saying that companies like Neuralink have promised that eventually these procedures whenever they're ready for the average person to get one, are gonna be automated, like done by a robot, and they're gonna be incredibly safe. It's gonna be an outpatient procedure where you can go in in the morning, come out in the afternoon without needing anything extra, and apparently it's supposed to be completely reversible, right, which is awesome. With all that said, maybe the person that has a life-changing condition, if they get that implant, will be ready to sign up for it. But other people that might not need it for their everyday use cases would probably be more swayed by something that doesn't require an implant. For example, like me, right? I don't need an implant in my head, but if you tell me that I can put on a cap and start controlling robots, I'm gonna wanna try it out.
Daniel: Yeah, and my brain in these situations a lot jumps straight to my brother who's a firefighter. I'm like, what if he's somehow able to control an exoskeleton that helps keep him safe in a fire rescue using just the signals from his brain. And it doesn't require drilling a giant hole in his skull. So, I'm with you on that. And I think one of the other main hurdles right now for these more invasive methods, before we talk about the non-invasive methods, the invasive methods, the one that go inside the brain, that surgery is pretty risky and it does cost a lot too. So, in terms of commitment, in terms of risk, in terms of pure financial cost, it's pretty expensive to do the invasive BCI version, even though, so far, I think Neuralink has shown a lot of promise, right? They've shown pretty high-resolution control over computer cursor. Wasn't there something to note about like the first patient, didn't he just like play video games all night?
Farbod: He spent all night playing video games.
Daniel: Which like, again, shows that it was working well enough that he was willing to stay up all night and play video games, right? The problem being non-invasive BCIs, which are these brain computer interfaces, it kind of looks like a swim cap with a bunch of electrodes attached to it. And then again, it doesn't require any surgery. It basically just uses like ultrasound gel almost to try and improve connection of these electrodes to the brain and it's able to read the electromagnetic activity of the brain without any surgery. These are pretty interesting. They do a pretty good job at doing 3D mapping of activity in the brain, but they typically have struggled so far with getting accurate and consistent signals because essentially, they're trying to read the brain signals all the way through the skull making it hard to get high definition signals the same way that you would get if you were drilling into the skull and attaching electrodes straight to the brain. So, the benefit still looks like it's there from the non-invasive BCI side, but it's really hard to implement because the signals haven't been clear enough or as clear, let's say, as direct contact electrodes for people to be able to program and understand what exactly does the brain that's doing and be able to control a computer doing that.
Farbod: Right. And we were just talking about this during dinner. Elementary versions of this you might have seen in like a shopping mall where they would give you a headset, they'd tell you to concentrate and just by concentrating you could make a ball go up or something like that. And then over the years advancements have happened and this lab that we're talking about at Carnegie Mellon University in 2019, they were able to take it a step further and they were able to be the first group to have a robotic arm continuously track something but not great accuracy using a non-invasive brain computer interface.
Daniel: I mean, that's pretty impressive. And I just have a fun story there. It's like…
Farbod: Shoot!
Daniel: Like you were saying like the shopping mall thing, like you attached this thing to your brain and you basically, it was one dimensional control.
Farbod: Right.
Daniel: Lots of brain activity forces this ball to move up. No brain activity forces it to fall. For one of my science projects in middle school. We did this with one of these same like EEG type calves and we're just playing pong against a computer. But again, it's same principle, right? If you focus a lot, create a lot of brainwave activity, the way that I calibrated it for myself is like, if I like flex all the muscles in my legs, I would get a lot of brain activity and then it forced the pong paddle to go up. And then if I relax my legs and it comes down, it's a pretty cool trick, pretty fun. Cool for us to show off. I think we won like words or whatever, but again, that's not anywhere near as precise as you need to be. If your goal is to create three-dimensional control over a robot arm, or even to create two-dimensional control of a cursor on a computer screen, this was way too elementary. I promise you; I've played around with it. It's way too elementary to be able to say like, Oh, if you wake up tomorrow and you're paralyzed, this is the only way you have to communicate. I promise you, prior to this teams from Carnegie Mellon's introduction of this cool AI technology to help decode that, promise you you'd be really frustrated. Sometimes you couldn't even reliably win a game upon doing this.
Farbod: But I mean, it's funny you bring that up, because there's conventional methods of trying to understand and decode these brain signals. And then with the popularity of machine learning, the way this group was able to have their breakthrough in 2019 was actually by using neural networks, which allowed them to kind of pair certain electromagnetic behavior in the brain to the desired outcome over trial and error and gathering a large set of data, right? And that's what allowed them to have the breakthrough that they did, but they still hit kind of a ceiling of like, we can't really increase performance past this, we still need a lot of data, et cetera, et cetera. So that's where everything stopped in 2019. Now we're coming back to this group in 2024 and they have some news for us, something else to share. Do you want to jump into it?
Daniel: Well, I think they have refreshed their AI-based methods here. So, they're using deep learning, like you said, to train this AI model to understand what these EEG signals look like on the brain, these non-invasive BCIs and how to control a cursor. And they've done that a lot better than they've done in the past with refreshed deep learning methods. But in addition, they've also figured out how to leverage this learning and calibration from one person to improve the learning speed for this new model on someone else. So, I think those are both two very important discoveries there, they've not only improved the overall performance and the overall control, let's say if I were to use it a bunch of times and continue to train and recalibrate so that it could understand how my brain works, but it also has learned enough, let's say, meta lessons around how the human brain works and how the human brain waves work, that if I were to use this brain cap a lot and train this method or train this model that they have, and then you were to go take that brain cap and put it on, it's gonna learn a lot faster with you being the second person. And think of the economies of scale here. Once they get these on dozens and then hundreds and thousands of people, hopefully they get a really rigorous model that works like plug and play right out of the box, which would be super interesting.
Farbod: Yeah, you got economics tied into this too. But what I was going to say is, what was kind of in the back of my head is, what happened between 2019 and now that allowed them to like kind of break through this barrier that they were facing before and the answer is kind of right in front of your face, right? When I think about 2019, I think, I had heard of machine learning and AI and how it was going to be the future, but I didn't really, hadn't seen it really come to fruition. I look at the past two years and there was an absolute boom, both in terms of hardware to train massive data sets for different algorithms and whatnot, and what's become more open source and more available. So, this team even made a note of saying is, a lot of the methods they're using here, which was not available to them before, have come from computer vision and image processing. So, developments via machine learning and other fields have allowed them to take the same approaches and apply them to the medical realm or the great computer interface realm. And you're exactly on the money. What they've been able to do is tweak that model in a couple of different ways that has resulted in significantly better performance. Do you want to jump into the kind of performance gains they've been seeing or general trends?
Daniel: I want to like break down the secret sauce.
Farbod: Oh yes, we didn't even touch on the sauce.
Daniel: So, like in my mind, I feel like there are four main steps, let's say.
Farbod: Okay.
Daniel: Actually, maybe three main steps. They first selected like a number of different AI techniques, these deep learning models. One of them that they used, I think they developed on their own, proprietary, which is called EEG net, which is again, probably based off of this learning that they've had over the time period since 2019 to now, they said they've collected hundreds of hours of user data testing with these non-invasive BCIs on these EEG caps to understand how the brain performs, what they call it, a continuous tracking test. So, imagine you're trying to use your brain to control a robotic arm to follow a point that's moving around on the screen in front of you. So again, you're trying to continuously force this robotic arm to follow a ball that's bouncing around on the screen. So, they had this EEG net model that they developed and then they also pointed like you're saying to this open-source model called PointNet, which is basically used to help visualize differences between different locations of items in 3D space. And you can think about it this way. They're looking at different brain activity and then plotting where brain activity happens in 3D space. And then trying to use PointNet to correlate between these, if the upper left point on this point of the brain is really active in the lower right part of this brain is really active, create the, basically the vector between those two points in 3D space and then use that to translate what is the brain trying to do to control the computer. Those were the two main models I'm aware of that they mentioned in addition to the baseline model, which is basically the state of the art for today.
Farbod: Correct, and then…
Daniel: Excuse me.
Farbod: No, you're good. And then we take it a step further with transfer learning. Yeah. Right, and that was, I think, where it really piqued my interest, because they start talking about how, again, a big bottleneck with neural networks is that you need a lot of data. What transfer learning allows you to do is take a data set, let's say, from a session with Daniel, and then take that learning and apply it to Farbod. So, then you don't need a lot of sessions with Farbod to get that model working. You can transfer that model from Daniel to Farbod and still get a good enough performance.
Daniel: Yeah, and it's incredible, meaning that like, obviously with each incremental user, they're able to add more data and more performance to their deep learning model, but the idea being that, at some point you could reach some level of saturation. You've got enough user data that you feel like you've got a model here that's rigorous enough to do transfer learning to pretty much any user as they come up and their learning curve will be really steep and they'll learn really quickly. Imagine if, I think in this study they tested on 28 people, but imagine if they're able to test it on 280 people, then user number 281 with this new transfer learning model, this model doesn't have to learn from scratch how this person's brain works because it's learned from 280 other people's brains and it's able to get a head start on understanding how user 281, how their brain might work and it works a lot better for them.
Farbod: Well, I was gonna say that's the perfect segue into the last bit of the sauce, which is transfer learning and recalibration. Yeah. Which I mean, as the name implies, you basically take again, Daniel's training data, use it on Farbod, but obviously Farbod 's brain doesn't exactly work the same way as Daniel's, so there's gonna be some nuances, some differences there. So, what you end up doing is you use that transfer data as a starting point. And as the sessions are ongoing, you have the model recalibrate to work with the way that Farbod’s brain as well.
Daniel: And I'm not sure exactly what the recalibration tasks are.
Farbod: I'm guessing it's a reinforcement learning process that allows you to say it has some sort of reward behavior that says, like you were mentioning earlier, activate left. Is Farbod activation of left matching with what we expect from Daniel's transfer method.
Daniel: Yeah, I mean, that's almost what I imagined it would be like. It's like, you know, when you're trying to like set up custom shortcuts on a keyboard, or custom controls for video game, and it's like allows you to record your keystrokes. And it's like, oh, like to make my character jump really high, this is the keystroke that I want to record. I wonder if it's pretty similar to that where it's just like, oh, like, we're going to tell you to, you know, in your brain control this thing to move left. And it captures what brain waves happens. I'm not sure if that's part of the recalibration, but the parallel I drew there in my mind right away was like, oh, like setting up video game custom controls. Cause this is like, ultimately that's what this is, is like using your brain as the ultimate custom control where you're not hitting keys, you're just thinking about the ball, tracking the ball and you're doing it correctly. I thought this was really interesting. And then one extra interesting part of the secret sauce, something that was a little hard for us to digest. We sat here and kicked it around for a little while to just understand exactly how it worked. But they use this metric called normalized mean square error, which basically helps them to quantify how close the cursor can get to the target controlled by their participants' thoughts. So, in a little bit, when we talk about the so what, we talk about the significance of how well these models performed. This is how they measured it. Essentially, how close the cursor can get to the target. What was the error between where the cursor should be and where it actually ended up? That's how they measured the performance of these different models versus the baseline current state-of-the-art, essentially measuring how close were you to the mark trying to control this robotic arm to track the dot on the screen. I thought that was pretty interesting, as in their key metric here is just how close can you get to the target.
Farbod: It's like the variance pretty much at that point.
Daniel: And it's not, you know, they did focus some on lag.
Farbod: But then, I think across the board, they did lag adjustment. So, you could just purely look at how the model's doing.
Daniel: And I thought that was pretty unique as well, that they developed basically this error measurement model to compare current state of the art, this model, this model, this model, to find out what was best.
Farbod: Do you want to get into the so what?
Daniel: Yeah, let's do that. So, I think the big headliner here is that they were basically on a relative basis able to reduce error by about 25% in a single user setting after seven trials. So, this means if I were to sit there and use their EEG net model, which is the one that they developed, after seven trials of me trying to play this game to use the robotic arm to track the ball bouncing around on the screen and this continuous tracking task, the amount of error would be reduced by about 25% after just seven times of me trying the game. Compared to the current state of the art, this is the best on the market, this is the software that you should be purchasing that comes along with these EEG caps, they're able to get 25% better. But the part that I thought was really, really interesting is the transfer learning portion of that. So, if you remember, they're doing multiple repeated trials on a group of people to try and build this reinforcement learning model, and then the transfer learning portion, which was how does incremental user n+1, how well does it perform for them? And one thing that was really interesting I saw is they were able to achieve similar performance as they did in the single user setting after seven trials. When they did transfer learning, they were able to get similar performance within only four trials. So basically, this means like for each incremental user, for each incremental tranche of users that they onboard, if this trend continues, it gets better and better at learning faster and people achieve better and better performance within a shorter period of time with less training rounds. And if you think about this from a user adoption perspective, people are gonna be less frustrated with the first couple times they use this because it's gonna feel more like magic. It's gonna feel more like it starts working right away.
Farbod: And then one thing that I thought was funny is that with the conventional approach, at the first session, it's almost always performing better than any of these models. And then by the second session, you just immediately see an improvement in all of the rest of them, except the conventional one. The conventional one goes up, goes down sometimes because it's not improving or degrading.
Daniel: It's the normal variance, right?
Farbod: Exactly, it's just kind of like a normal variance, whereas the other ones constantly get better until they converge on the best that they can do. So, these folks have obviously proved that they did not hit a ceiling, that there is more room to grow here, and they've shown that wiggle room in two different fronts. The last thing that I wanted to say is, in addition to the transfer learning, improving over four sessions, they even added the trend for transfer learning plus recalibration and that added even another extra layer of improvement. So, I don't know, the future is bright. Imagine they kept going on that trend for another three more sessions. Seven sessions here, seven sessions there.
Daniel: No, I agree and I think one thing that's really interesting here, outside of the full using this EEG for brain computer interfaces, right, for people to control robots and a cursor on a screen, et cetera, which obviously that's really noble cause on its own. They also mentioned that this development could lead to better understanding of how the brain works for other types of disabilities as well. One of the things that they mentioned is epilepsy. For certain types of treatments for epilepsy, you want to do brain stimulation in the brain, but you don't know exactly which part of the brain is the one that really needs the most attention. And by using this BCI technology with their updated algorithms, they can quickly learn and hone in on which portion of the brain is misfiring during an epilepsy attack and like during a seizure or another type of episode. Basically, it's the same principle, you're trying to understand exactly which parts of the brain are firing during a certain type of neural event. In this case, it's not controlling a robotic arm, it's during a seizure, but they're able to quickly hone in on which portions of the brain are impacted in a much better way than you can do so far today.
Farbod: Yeah. And again, I'm going to go back to what I was saying earlier. The average patient who might be suffering from a brain disorder might not be ready to commit to a hold on brain implant. One of our friends from college suffers from epilepsy, but it's not frequent enough for them to want to commit to an entire implant. But going to a doctor's office, occasionally doing these scans and being able to have targeted treatment, that seems like a much more feasible solution, again, for the average person. So, I'm really excited to see that not everyone has committed to implantable brain computer interfaces are the only way in the future that there are alternatives that are getting attention. Yeah, with that said do you want to do the TLDR?
Daniel: Yeah. All right. I mean honestly guys; I think this research here is gonna be the reason why you don't need to get a whole drilled in the side of your head and a computer implanted to be able to control a computer using your brain. So, I know Elon Musk, Elon Musk is launching something really cool with Neuralink and it's made a lot of headlines recently but this team from Carnegie Mellon University is using non-invasive brain computer interfaces. So, the type that just sits on top of your head without having to be implanted, they're using machine learning to improve these non-invasive brain computer interfaces to the point where they're able to accurately read brain signals through the skull and get really improved performance in moving a cursor around a screen, doing basic computer tasks, but only using your brain to control the computer instead of a cursor and keyboard in front of you. They used a lot of deep learning models and developed their updated model that even allows it to have improved transfer learning, meaning that if I train the model a lot, when you put the cap on it, it works really, really well for you as well. And again, I think this is really interesting. And honestly, that's why I don't know that we'll need a neuro link implant in the brain. Maybe this is the future here when we can all put on a cap, you know, while we're doing certain tasks at work and then take it off when we don't need it. I mentioned my brother earlier as a firefighter, but I also think on construction sites. It'd be really interesting to have something like this embedded in the hard hat. Then you're able to control the crane to, to move material around. I don't know. I feel like the opportunities are endless. We've talked a lot about cobots, collaborative robotics. This is the potential control method for collaborative robotics and all sorts of new types of technology.
Farbod: You know, I want to hear from our audience. I think we can do polls on a Spotify episode.
Daniel: I think so.
Farbod: Right. I want to know who wants an implant and who wants a cap. I'm curious.
Daniel: I'll take a cap.
Farbod: I'll take a cap. I'll sign up for the cap experiments. Not for the chip ones though. I don't know.
Daniel: You seem like an early adopter of.
Farbod: Eh. I'll pass on anything that needs to get implanted into me.
Daniel: Fair enough.
Farbod: I think that's it. I don't think we have anyone to thank this week, do we? I mean, besides our lovely audience, which we thank every week.
Daniel: Yeah, I just, I wanna mention, here's our passion plug moment.
Farbod: Plug it.
Daniel: We're launching a newsletter. We would appreciate everyone's support in signing up for that newsletter, trusting us with your email. We know it's not a trivial thing to trust someone through email. We promise we're not gonna share your email with anyone. We're not even gonna take up space in your inbox unless we think it's a true golden nugget, valuable golden nugget. Essentially what we're doing is gonna be distilling all the interesting and impactful technology that we see on the podcast into a short digestible format that's easy for you to skim, you know, maybe you got two, three minutes on the toilet. You can skim this newsletter really, really easily and clean up all the awesome insights that we're learning at the forefront of technology in the written format, as well as the audio format, because you're already listening to us on the pod.
Farbod: You love it, or we'll give you your two minutes back. Promise.
Daniel: And we'll never email you again if you don't like it.
Farbod: This is true, this is true. With that said, folks, thank you so much for listening. As always, we'll catch you in the next one.
Daniel: Peace.
As always, you can find these and other interesting & impactful engineering articles on Wevolver.com.
To learn more about this show, please visit our shows page. By following the page, you will get automatic updates by email when a new show is published. Be sure to give us a follow and review on Apple podcasts, Spotify, and most of your favorite podcast platforms!
--
The Next Byte: We're two engineers on a mission to simplify complex science & technology, making it easy to understand. In each episode of our show, we dive into world-changing tech (such as AI, robotics, 3D printing, IoT, & much more), all while keeping it entertaining & engaging along the way.